Document Type
Conference Proceeding
Date of Original Version
2017
Abstract
The increasing use of wearables in smart telehealth system led to the generation of large medical big data. Cloud and fog services leverage these data for assisting clinical procedures. IoT Healthcare has been benefited from this large pool of generated data. This paper suggests the use of low-resource machine learning on Fog devices kept close to wearables for smart telehealth. For traditional telecare systems, the signal processing and machine learning modules are deployed in the cloud that processes physiological data. This paper presents a Fog architecture that relied on unsupervised machine learning big data analysis for discovering patterns in physiological data. We developed a prototype using Intel Edison and Raspberry Pi that was tested on real-world pathological speech data from telemonitoring of patients with Parkinson's disease (PD). Proposed architecture employed machine learning for analysis of pathological speech data obtained from smart watches worn by the patients with PD. Results show that proposed architecture is promising for low-resource machine learning. It could be useful for other applications within wearable IoT for smart telehealth scenarios by translating machine learning approaches from the cloud backend to edge computing devices such as Fog.
Citation/Publisher Attribution
Borthakur, D., Dubey, H., Constant, N., Mahler, L., & Mankodiya, K. (2017, November 14-16). Smart fog: Fog computing framework for unsupervised clustering analytics in wearable Internet of Things. 2017 IEEE Global Conference on Signal and Information Processing (GlobalSIP), Montreal, QC, Canada. doi: 10.1109/GlobalSIP.2017.8308687
Available at: http://dx.doi.org/10.1109/GlobalSIP.2017.8308687
Comment
Debanjan Borthakur, Nicholas Constant and Kunal Mankodiya are from the Department of Electrical, Computer and Biomedical Engineering.
Leslie Mahler is from the Department of Communicative Disorders.
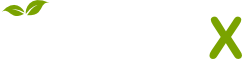
- Citations
- Citation Indexes: 81
- Usage
- Downloads: 451
- Abstract Views: 22
- Captures
- Readers: 112
- Mentions
- News Mentions: 1
Terms of Use
This article is made available under the terms and conditions applicable
towards Open Access Policy Articles, as set forth in our Terms of Use.