Wind Turbine Fault Detection Using a Denoising Autoencoder with Temporal Information
Document Type
Article
Date of Original Version
2-1-2018
Abstract
Data-driven approaches have gained increasing interests in the fault detection of wind turbines (WTs) due to the difficulty in system modeling and the availability of sensor data. However, the nonlinearity of WTs, uncertainty of disturbances and measurement noise, and temporal dependence in time-series data still pose grand challenges to effective fault detection. To this end, this paper proposes a new fault detector based on a recently developed unsupervised learning method, denoising autoencoder (DAE), which offers the learning of robust nonlinear representations from data against noise and input fluctuation. A DAE is used to build a robust multivariate reconstruction model on raw time-series data from multiple sensors, and then, the reconstruction error of the DAE trained with normal data is analyzed for fault detection. In addition, we apply the sliding-window technique to consider temporal information inherent in time-series data by including the current and past information within a small time window. A key advantage of the proposed approach is the ability to capture the nonlinear correlations among multiple sensor variables and the temporal dependence of each sensor variable simultaneously, which significantly enhanced the fault detection performance. Simulated data from a generic WT benchmark and field supervisory control and data acquisition data from a real wind farm are used to evaluate the proposed approach. The results of two case studies demonstrate the effectiveness and advantages of our proposed approach.
Publication Title, e.g., Journal
IEEE/ASME Transactions on Mechatronics
Volume
23
Issue
1
Citation/Publisher Attribution
Jiang, Guoqian, Ping Xie, Haibo He, and Jun Yan. "Wind Turbine Fault Detection Using a Denoising Autoencoder with Temporal Information." IEEE/ASME Transactions on Mechatronics 23, 1 (2018): 89-100. doi: 10.1109/TMECH.2017.2759301.
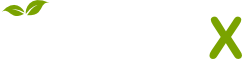
- Citations
- Citation Indexes: 255
- Patent Family Citations: 2
- Usage
- Abstract Views: 3
- Captures
- Readers: 147